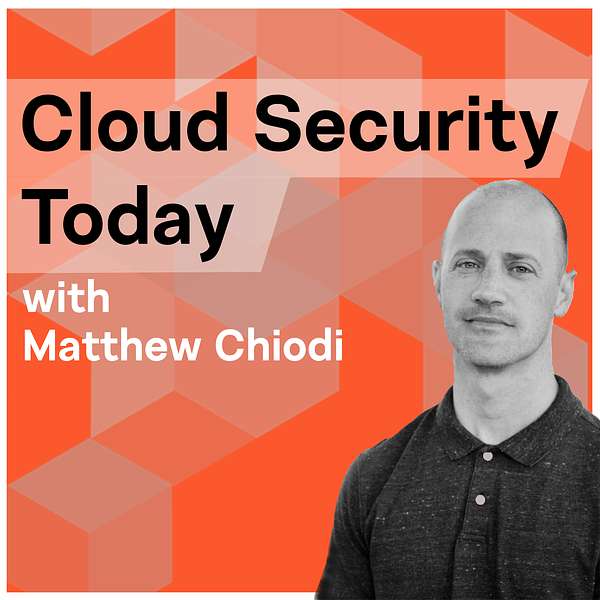
Cloud Security Today
The Cloud Security Today podcast features expert commentary and personal stories on the “how” side of cybersecurity. This is not a news program but rather a podcast that focuses on the practical side of launching a cloud security program, implementing DevSecOps, cyber leadership, and understanding the threats most impacting organizations today.
Cloud Security Today
The AI Episode
Episode Summary
In today’s episode, AI Safety Initiative Chair at Cloud Security Alliance, Caleb Sima, joins Matt to talk about some of the myths surrounding the quickly evolving world of AI. With two decades of experience in the cybersecurity industry, Caleb has held many high-level roles, including VP of Information Security at Databricks, CSO at Robinhood, Managing VP at CapitalOne, and Founder of both SPI Dynamics and Bluebox Security.
Today, Caleb talks about his inspiring career after dropping out of high school, dealing with imposter syndrome, and becoming the Chair of the CSA’s AI Safety Initiative. Is AI and Machine Learning the threat that we think it is? Hear about the different kinds of LLMs, the poisoning of LLMs, and how AI can be used to improve security.
Timestamp Segments
· [01:31] Why Caleb dropped out high school
· [06:16] Dealing with imposter syndrome.
· [11:43] The hype around AI and Machine Learning.
· [14:55] AI 101 terminology.
· [17:42] Open source LLMs.
· [20:31] Where to start as a security practitioner.
· [24:46] What risks should people be thinking about?
· [28:24] Taking advantage of AI in cybersecurity.
· [32:32] How AI will affect different SOC functions.
· [35:00] Is it too late to get involved?
· [36:29] CSA’s AI Safety Initiative.
· [38:52] What’s next?
Notable Quotes
· “There is no way this thing is not going to change the world.”
· “The benefit that you're going to get out of LLMs internally is going to be phenomenal.”
· “It doesn't matter whether you get in now or in six months.”
Relevant Links
LinkedIn: Caleb Sima
Resources:
Skipping College Pays Off For Few Teen Techies
The future of cloud security.Simplify cloud security with Prisma Cloud, the Code to Cloud platform powered by Precision AI.
Disclaimer: This post contains affiliate links. If you make a purchase, I may receive a commission at no extra cost to you.
**Auto transcribed, expect weird stuff not actually said by the guest or host**
Podcast Open: This is the Cloud Security Today podcast, where leaders learn how to get Cloud security done. And now, your host, Matt Chiodi.
[00:13] Matt Chiodi: Artificial Intelligence. Oh, the topic that strikes fear into the hearts of so many people. But don't worry. On today's podcast, we have Caleb Sima, and he is going to dispel some of the myths around artificial intelligence. He's going to talk about what's real, what's not reality, what's FUD, and some of the attacks that are happening very quickly as we learn about AI. Now, for those of you who don't know Caleb, he has been around the industry for many years and he's got a really interesting background and story that I won't spill the beans on here because I want you to hear it in his own words, but if you are somebody who maybe did not follow a traditional career path - maybe you didn't go to college and you feel like cybersecurity is one of those fields that you could never get into without having a college degree, you need to listen, because Caleb's story, especially when you hear about his family background, I find it highly inspiring.
So, enjoy the podcast. This is a good one. Get ready to learn about artificial intelligence and how it's going to impact you in the future.
Caleb, thanks for coming on the show.
[01:29] Caleb Sima: Thanks for having me.
[01:30] Matt: Alright. This is going to be fun. So, in researching, I always check what's the background of the guests. What's their career trajectory? For you, this was super interesting. So, I found this article from 2004, from NBC News, and it was titled “Skipping College Pays Off for Few Teen Techies.” So, you were profiled at the tender age of 24, and you were already the CTO at SPI Dynamics. Is that how you say it? SPI Dynamics.
[02:02] Caleb: SPI Dynamics, that's right.
[02:04] Matt: So, I had to immediately ask, what led you to not only skip college, but actually drop out of high school at the age of 16? What did you see coming that, maybe, others didn't?
[02:18] Caleb: Wow, you really dug pretty far. That's a really old school article. To answer your question, it's not that I saw something or was genius in what was coming. It's funny, what led me to drop out of high school, I dropped out ninth grade, high school, basically.
[02:43] Matt: So, you weren't even 16? How old were you?
[02:45] Caleb: Yeah, I was 16. It all had to do with me running away from home. I had, at the time, a lot of challenges in family life. Specifically, I would get in trouble a lot in school and my stepdad, at the time, had said, “you know what? I'm going to put you on restriction where you can't touch or read anything about computers for a year,” and I was like, “I'm not going to do that,” I ran away from home, I ended up crashing at my best friend's house where his dad let me do this, and I never went back. I got kicked out of multiple high schools during that timeframe. In ninth grade, I got kicked out of four different high schools – two in Atlanta, two in Florida. I remember. I just was like, “I'm not going to go back,” and I decided I just wanted to work and only read about computers, at the time. That's what mattered to me, so I didn't drop out of school to go become a founder of a company. I dropped out of school because, I liked school, I was just not a good fit, and I ran away from home, had nothing else to do, and I didn't want to go back. So, that's where it ended up being, and then, lots of things happened during that timeframe where I ended up starting that company, but there's a lot of incidents before that. So, there's no forecasting. It's not like some brilliant move. It just happens to be chance, and a lot of luck, too.
[04:45] Matt: So, I've got to ask you this as a parent of a child that, at least one of my children, who does not like school at all – was your path in school up to that ninth grade, did you always not like school, or did you find that the format didn't fit? What was that like?
[05:03] Caleb: You know, I just never really did. I struggled in school. I generally, for most of the time, got bad grades. It was hard for me to go to school. I was an adolescent. Now that I'm an adult, if I look back and think about where I was, as a kid, I was a really tough kid to deal with. I was very rebellious, I was always getting in trouble, and it was really easy. Basically, if you wanted me to do something, I would just do the opposite. So, you could play some reverse psychology, and I would just reverse you out of that. I was a really frustrating kid at the time, and as you know, teachers and other academic places just didn't want to put up with that, and I don't blame them. By the way, when I think back on my behavior, it was pretty rough, but it's hard for me to go through academics, and I never was very good at it. I never had an interest in it. It just bored the hell out of me.
[06:16] Matt: So, let's skip forward a couple years. You sold your company SPI Dynamics to HP in 2007, and then you've got a litany of just building companies, selling them. I saw that you did a stint at Andreessen Horowitz, or a16z, as an entrepreneur in residence. So clearly, at least for you, not going to college, or even finishing high school, has impacted your ability to succeed in what I would call a top 1% way. Now, society would tell us that you're at a major disadvantage, because you didn't finish high school or college, and I'm guessing that specifically when you were at a16z, you were probably surrounded by a lot of MBAs. I don't want to say that you did, but did you have to deal with impostor syndrome? Did you deal with that? How did you approach that? Because obviously, I’d imagine, you came from an extremely different background, even just academically than probably 99% of the people that you were interacting with. What was that like?
[07:19] Caleb: Yeah, I mean, I will say, it's really tough. I was always the youngest in the room, and I always was treated that way. So, there's an aspect of saying, “well, if I'm always the youngest in the room, how do I ensure that my views are held important, or that I can be at an equal level with people who were, at the time, 20 years-plus older than me?” In order to do that, I just had to be good at my job. I had to know my subject matter. I had to understand what it was, and I had to be better, and so, I had to fight and dig pretty hard to continuously learn and continuously fight, because in a lot of my growing up, in that scenario, I would get pushed down quite a bit. People would talk down to me, people would treat me like a child. There was definitely plenty of that, that I had to go against.
[08:34] Matt: Although, I think I've told you this story at least once before, but I remember actually watching you on stage at a Black Hat event sometime in the early 2000s, and I remember, at the time, you were really young, and you were on stage. I think it was a debate. It was a debate. I was trying to find it, but I can't. There's a debate that you did with someone who was at least 20 some years older than you, and I remember watching, at the time, being like, “this is awesome,” because you were clearly far more knowledgeable. I don't remember the topic, but I remember thinking, “this guy knows his topic.” Here's an older gentleman, who quite frankly, he’s probably my age, now that I think about it in reverse. Somebody who’s not young anymore. Anyway, I just remember being impressed by how well you knew the topic, and it was a heated topic. Again, I don’t remember what it was, but I can still see you on stage, in my mind. I remember watching you, so absolutely, kudos to you. I had no idea what your background was at the time, but it was clear that you didn't just get angry and have an emotional response. You had very articulate, very well-informed views on the topic, and the audience was definitely, let's just say, in your favor. So, congrats.
[09:44] Caleb: It's hard. It's a hard thing to do, to do that. I will state one thing, now that I'm the older person, and the one thing you also notice is, at the time, I was unusual, but I actually think today, I'm not. When you look at kids who are 16/18 years old, they are far smarter than even I was, in technology, and even in cybersecurity, at the time. These kids are growing up in cybersecurity, in technology, in computers, where when I was doing it, it was very unusual. I remember, I would say, because I was into the security stuff, I might ask my parents, “could I in get a job in security?” and they were like, “you don't want a job in security. It doesn't pay very well,” because they're thinking security guards. So, these kids are brilliant. I talked to kids who are just going into college or coming out of college, and they are freaking amazing. You talk to them, and I'm like, “wow.” You'll never be able to keep up with the level of smarts coming out of the kids these days. Going back, also, by the way, to your imposter syndrome – never stops. There's always imposter syndrome.
[11:09] Matt: So, you talk about imposter syndrome, about kids coming up now that, one of the things that “kids” that are coming into the market now, whether they're still in high school, college, or they graduated college, one of the things that they're coming up against, that you and I did not have to deal with was AI. It was something that some weird professor that I had talked about, some theoretical thing that I thought, “whatever. Sounds like a movie.” So, today is very different than when you and I were first coming into the market. The biggest thing that I think everyone has been talking about is ChatGPT. Of course, that's the one that's got the most name recognition. I specifically wanted to have you on the show, yes, because you have a super interesting background, but I saw your LinkedIn posts over the last couple of months, and it really intrigued me because I saw that you were becoming a student. I know that you don't have an AI background, you don't have a PhD, and all that kind of stuff. So, you've seen a lot of tech that has been hyped over the last 20 years. Help us maybe separate, a little bit, fact from fiction when it comes to AI. What is so impressive, and what's different, specifically from all the machine learning hype that we heard about, maybe, even just three years ago?
[12:28] Caleb: Yeah, I mean, there's a lot to your question, but I would say, being in security and cybersecurity my whole life, I feel very comfortable with that sort of subject matter, and in fact, even prior to Robinhood, I was at Databricks, and Databricks is a well-known AI machine learning company, per se, and at the time, I had started to get caught up a little bit in the subject matter when I was at Databricks, and it was fascinating around how machine learning works. How do you make this thing actually do things that are productive? And I really was interested, but at the time, just never really got the chance to go and dig in after I went to Robinhood, and then when ChatGPT really made its big splash, and that really helped create, between ChatGPT, Midjourney and Stable Diffusion, and the Gen-AI artist and image things, it just created this wave that came, and you started looking at this, and I was like, “there is no way this thing is not going to change the world,” and I looked at it when Cloud first came and made its appearance.
This is exactly similar, except this is probably much, much bigger, and so I decided, “hey, I have got to learn about this because I've got to understand how it's going to affect me, my decisions, how it's going to affect cybersecurity, how do I use this to help solve security problems?” so, I decided I'm just going to be a student for, what you said, Matt, and I'm going to just dig down and learn, so I started spending almost full-time, left Robinhood, started focusing nine-to-four, almost like a job, learning and understanding, and playing with anything I could on understanding AI and LLMs, and everything I could, and it's been a really phenomenal journey. Obviously, like any deep subject, the more you learn, the more you learn you don't know. So, I am by far not an AI expert, by any means, but it's been a lot of fun.
[14:53] Matt: It's awesome. Now, let's just go to the basics. I don't want to assume everyone in the audience knows some of the basics of AI. You mentioned the term “LLM.” What's an LLM? If I'm using ChatGPT, am I interacting with an LLM? Help me just break some of these AI 101 topics down.
[15:10] Caleb: Yeah, actually, I just got finished doing a sort of 101 on LLMs presentation that I'm giving for Cloud Security Alliance, so I guess this is fresh in my head. The first thing I would say, in my journey in this, one of the first things that confused me were the terms. You hear “Gen AI, AGI, deep learning, supervised learning.” Where does all of this sort of layout?
So, the first thing I'd say is just, as a really simple example, AI is a goal. So, you think of AI as the goal to go to. Can we get to AI, artificial intelligence? There are three levels of artificial intelligence. There's Artificial Narrow Intelligence, Artificial General Intelligence, and Artificial Super Intelligence. So, you'll hear these terms as an AI, AGI, ASI. I think of it like growing up. Artificial Narrow Intelligence is, you're a kid. Artificial General Intelligence, you can reason, to think like an adult. Artificial Super Intelligence is basically aliens from outer space that are way smarter than me. So, those are the three stages, and I think underneath that, machine learning is the way to get to AI, so under machine learning, you have all these different methods – deep learning, supervised learning, self-supervised learning, all of these different kinds of things that are there, that are being used today to create Gen AI, or Generational Artificial Intelligence, which is what we see in ChatGPT, with LLM, Large Language Models. It produces output, or like Midjourney, or Stable Diffusion, they create images or create art. You can create audio, you can create music, all of these things are creating. That's the Gen AI part, and that's underneath that, and it uses all these techniques in order to create that,
[17:03] Matt: Love that. So, one of the things I shared with you before the show was something that popped up in one of the newsletters that I follow, and that was the LLM attacks, and I'll put this in the show notes, but if you go to llm-attacks.org, it goes through, I wouldn't say it's theoretical, because I think they actually did it, it's not theoretical. So, you can take some special characters, and put it in with a prompt, and you can get LLM to do things that normally they would be patched not to do. Now, one of the things that caught my interest, when I was reading just the really brief summary was, they made a differentiation between open source LLMs, and I guess those that are not. Help us to understand maybe just that a little bit. What is an open source LLM? Everyone's familiar with ChatGPT, Bard, and those other ones, but what is the open source?
[17:59] Caleb: Yeah, so you've got open source LLMs, like LLaMA, Orca, there's a bunch of these ones that are created and are open sourced. So, for example, they go, and they build all of the training data, and it takes, for these really large LLMs remodels, these things are billions of parameters, so from 7 billion at the small, 70 billion at some of the largest, and in order to train something at that size, it requires a huge amount of cost and compute, so companies like Facebook, oddly enough, are the ones who are really, really moving forward the open source area of this field, because they are using their compute, and then releasing all of the information and all the models for free to use, so this is really pushing ahead a lot of the open source community because you don't want AI in a field that is closed by just OpenAI, or just by Google. You want an ability to be able to say, “there's lots of different models that you can use yourself.”
I'll give you a good example. When you use something like OpenAI, it's very filtered, there's a lot of safety mechanisms that are put in place, a lot of content that you may not be able to reach, which is good for general consumers, but let's say, for example, we're in cybersecurity, so one of the things that we want to query in something like an LLM to get information or thoughts around is going to be the dark side of the web, like what is going on in 4chan, and all of the discussions in 4chan you may want access to, but clearly is way more dangerous for any general LM, so they will train that out, so that 4chan data is not going to be produced, which is considered “not polite.” So, you want these models that you can have untrained, or have the ability to get the information you want, so these open-source models are out there to do that.
[20:08] Matt: So, the open source, is that the code? Or is that the datasets? Or is it both? Like what does that actually refer to?
[20:17] Caleb: It refers to the model itself and the code in order to interact with it. I don't know if the datasets are. That's a good question. I'm not familiar if that is actually available as well.
[20:31] Matt: If I'm a security practitioner, pretty much in any industry, I've obviously heard about AI at this point, and maybe I've even been asked to look into it to secure it, or some companies have even attempted to block access to it, partially because there's privacy and security concerns with if I'm pasting sensitive data into OpenAI, are they grabbing from it, but I guess that, from a security practitioner perspective, where should I start? What method or processes would you use to approach something like this? Again, practitioner, maybe they've been asked to look into this for the company, maybe they want to start developing some policies around the use. Where would they start?
[21:15] Caleb: Yep. Now, this is the hottest topic, obviously, in our industry, but I think the first thing you need to say is, “where are LLMs specifically being used?” Because that's what we're talking about. There's also machine learning AI in general, which has been used in a lot of different places, a lot of different ways, but I think most people are concerned about LLMs. So, first, where is it being used, for example, in your enterprise? Are you worried about your employees just sending data to OpenAI? Is that your primary concern? Or is your primary concern that your company is building a product that is using an LLM as a feature, and you need to figure out how to make sure that that LLM is safe for your customers to use? Or is it that your employees are using some LLM internal to your company that has and consumes internal private data, which can be useful for internal employees? So, it's really sort of these three areas that LLM 's are used in an organization.
So, as a security practitioner, you have to think about, “what am I most concerned about? What is my company doing? Am I doing all three of these, which is pretty rare today, or am I only worried about employees sending their stuff out?” And then based off of that, then you start figuring out what to go do.
Let me give you an example. Today, I think the biggest worry when I sit down at these dinners, is you hear about CISOs shutting off OpenAI access and blocking it completely, and absolutely banning all employees from using OpenAI. Why? Because of this fear – the fear is they're going to take all this confidential data, they're going to shove it into OpenAI, and they're going to ask questions about that data, and therefore, that data then goes to OpenAI, they'll take it, and then they'll reuse it in their training model, so that some attacker can then extract that data about their company out through that LLM. This is the fear. That by far, I would say, that is what you hear about most, and for this specific thing, I absolutely think it's a low risk.
LLMs don't work this way. A lot of people think it's a data store. You throw a bunch of data in there, there's a training data, that's a data store, and then you just ask somebody, and they directly take that data and then spit it back out. It doesn't work like that. LLMs are generators. They work off prediction. So, it's all about what word is most likely to follow the next word, and that's how they work, and so it's really low risk to be able to go and say, “hey, I have a social security number that went over to OpenAI. Can some attacker now extract that social security number?” Good luck. In fact, I wrote a LinkedIn post specifically saying that this issue is overhyped and is low risk, and explained my thought process around how extracting very specific types of data is extremely difficult to be able to do out of your big LLMs like OpenAI and what they do. To me, I actually think you have a bigger risk in what your employees paste into Google search boxes, stuff into Stack Overflow, paste into Slack channels of other people. All of your data, that stuff is going out in way more places than OpenAI being that risk and some attacker pulling it out of an LLM.
[24:46] Matt: Outside of, as you mentioned, Slack and some of these other ones, and I agree with you, what if we can confine the risks to LLMs, to AI, what maybe are some of those risks that you think people aren't thinking about today? Because you're right, that's the one that everyone's talking about the most. I think there was a memo from, I think it was Amazon's general counsel, a couple months back saying, “some of the responses we're getting out of OpenAI look like some sensitive internal data.” That concern aside, is there something else that you think people should be more concerned with, specifically, around LLMs?
[25:27] Caleb: I think that where the issue becomes really interesting, first, I'll start from a practitioner perspective, is when your companies internally start using LLM for your employees, and let's be clear, that's going to happen, because the benefit that you're going to get out of LLMs internally is going to be phenomenal. Like taking Gen AI technology and you just think about how internal search engines work today, which are very bad, and how you gain information about your company and about processes in your company. It's extraordinarily static, old, and very badly used, versus take, for example, many companies have internal wiki pages, or confluence pages. They're always out of date.
Think about a world where LLMs could actually auto-update these things with current information and do it in a way that makes sense. That becomes a phenomenal bit of productivity boost. I think those kinds of LLMs, and how you poison those LLMs, and how you prompt inject those LLMs, and how you make those things do bad behaviors, is going to be a really interesting scenario that will happen later. I also think being able to protect against the kinds of information that LLMs actually generates out is going to be fairly difficult.
So, I'll give you an example. If you are an engineer inside of your company, and you go to your company chatbot, and you're like, “company chatbot, I want you to give me all the salaries of the employees inside of the company.” How, today, does the LLM know not to give you? Or how does that work? I think that is going to be a scenario that we're just now starting to get a lot of focus on, is identity, Alt-Z, how do you do the proper access control around roles, all of this starts becoming a really big issue when you start talking about deploying these internals in our enterprise. Data poisoning becomes really interesting, because now I can just create up a Word Doc that maybe says the CEO is an idiot 50,000 times and then in the chat bot, when they go tell me about the CEO is going to be like, “the CEO’s an idiot.” So, when you start making the datasets small inside of enterprises and companies, and then that information gets regurgitated out, then these attacks become a lot more interesting, too.
[27:59] Matt: Yeah, I think you're right. It's funny, you mentioned wikis and stuff like that. I'm just thinking of all the large companies I worked for over the years that had some kind of intranet. Intranet, some of them listening may not know what that is, but usually have some kind of intranet, and you go there occasionally trying to find maybe some benefit information, and it's always old, it’s always out of date, so I know exactly what you're talking about. So, I guess from my perspective, that's the one side of AI. The other side of it is how a security practitioner or how a security team may actually be able to take advantage of AI. So, I guess my question for you is, so many vendors say that they've got AI in their products. I mean, you go to RSA earlier this year, everything was AI. Help us a little bit to determine what's real, and are there practical applications of AI in cybersecurity today?
[29:02] Caleb: Yeah, actually, that is what interests me the most. I sort of see two buckets. I see bucket one being “how do I protect AI and secure AI?” And then bucket two is “how do I use AI to solve fundamental security problems better?” And that bucket is the bucket I'm most interested in. I'm less interested in the security of AI, and more, how do I use it? And when you look at that, I think the definition of AI is interesting, because, as all marketing people do, you can say it's AI anywhere if you have some machine learning model implemented in your product, which by the way, products that have machine learning models, implemented it for years, decades, there's been “machine learning models” in products, and so them just calling it AI is just the new buzzword of being able to say it's AI, but in reality, we've not hit AI, we've not hit Artificial General Intelligence. Remember, AI is a goal. So, when you see a product that says, “we use AI,” it means that they have some machine learning models in there that might help make your job easier. So, that's not really AI, but I will say, I think that this is super practical, and I think there's a lot of really amazing opportunities around helping do security better.
Let me give you an example that has almost nothing to do with security but is a phenomenal thing that I knew AI was going to be good for. When you're a CISO, and you look at your team, and by the way, this applies to any team, but CISO specifically, project management is a huge pain. When you have multiple different things around KPIs, OKRs, whatever you want to call them, around “how do I make sure that my enterprise is more secure? What teams are doing what? How are they doing it? How are we getting better and closer to our goal?” A lot of that requires these people inputting reports, keeping track of what's going on, and 50% of your cybersecurity team is wasted, updating status reports, JIRA tickets, all generating reports for me, the CISO, to look at and say, “looks like you're doing good.” It's a huge waste of time to go and do this, but there's a company that I just saw a pitch on for cybersecurity for CISOs specifically, that uses ML to go and actually automate a lot of this stuff, so it will look at your JIRA projects or KPIs, or OKRs, look at the status of where you are from a security product area and pull all that in and start summing up and keeping track so that people don't have to individually do this. I saw this demo and I was like, “that is something that you can only do because of LLMs.” You could not do this a year ago, so seeing something that would save a tremendous amount of time, just from a management status, project perspective, is going to add tremendous value to our teams, because they can now focus on doing real work, and that kind of thing just wasn't doable a year ago. So, I'm really excited about the things that you're going to see in two years, three years from now. It's going to be phenomenal.
[32:32] Matt: What do you see as maybe some areas, if we think about the SOC, if we think about incident response, think about GRC, just some of the different functions that typically exist within a cybersecurity team, are there other certain functions that you think will be more impacted than others with AI over the next two to three years?
[33:00] Caleb: Yeah, obviously, I think the most obvious answer to this is going to be the SOC. Why? Because, generally, you have tiers of SOC, of “I'm a level one. What I really look at is just seeing if there are any critical alerts and then to do some investigation, or does one plus one equal two?” I think that's going to make a big change. Let me give you an example. There's a startup company called Enzym.ai, and their focus is not to replace the analyst in a SOC, but to be able to create a helper in the SOC, where it acts very much like an analyst, where you can do things that says, “you're a junior person, go grab this data, that data, and that data, and then tell me what the sum-up of it is,” and it will go and it has access to this thing and says, “I notice that from this machine to that machine, I saw lateral movement, but I also saw an exploit that happened here. This is what this looked like, and here are the log excerpts that I was able to pull back,” and seeing that kind of thing get created is, again, it's phenomenal. You'd never be able to do this with scripting or code a year ago. This kind of thing can only be done through this capability and watching how you interact with this thing. Basically, it's like Siri, but for a SOC analyst and a detection team, and being able to say, “go do this, grab this, give me an analysis of this,” and it just really produces great output is pretty phenomenal. Again, it's really exciting, and this is the stuff that's only happening now. Matt, one thing, I want to be clear, this wave, I don't think, has really taken off, except maybe, about a year and a half ago, so think of how this in two years, it's going to be nuts, all the new stuff that's coming out.
[35:00] Matt: So, it's not too late? So, if someone's thinking like, “man, I'm really far behind,” It's not too late to get in and start to learn and get involved with this?
[35:09] Caleb: No. In fact, as part of my process and learning, one of the things I did learn is that this thing moves so fast. The state of AI in general is so quick and so fast. Literally, what you learn today as a fundamental and how things work will change next week, and then everything that I learned today is just obsolete. One of the things is, it's actually good. One of my things is, to an entrepreneur, or someone learning the space, I would wait, because you need to wait to see this thing settled down a little bit. It's like standing on quicksand. There's just so much movement. There's no solid ground of what is truth and what is not, because things are changing so rapidly in this field, and at some point, within another six months or a year, there are going to be these foundations, “this is now how these things get done,” and then you're going to be able to start building on those foundations, so I would even say, it doesn't matter whether you get in now or get in in six months because if you get in six months, you actually might have a better advantage, because you're going to be learning about things instead of wasting your time on all of these different paths that actually ended up not that.
[36:29] Matt: So, you were recently named the chair for AI safety initiative by the Cloud Security Alliance. Tell us a little about the role and some of the expected deliverables or outcomes.
[36:40] Caleb: Yeah, so, obviously, when you think of Cloud and you think of AI, there are a lot of comparables. When the Cloud first came out, there was a lot of fear about what does this mean? What does this do? What jobs does it take? A lot of, “how do we secure this? How do we make it safe? Like what do we do about it? How do we message and talk to the board or to anyone around the risks around Cloud and AI?” These are all exactly the same that's happening now with AI. Those exact same terms, you can just replace it and it's exactly that same pattern with AI, and I think Cloud Security Alliance is saying they're getting asked by a lot of people around “how do we solve the exact same issues and fears we have with Cloud, we are now having with AI? What does that look like for us? Help us,” and Jim Reavis, who is the founder of Cloud Security Alliance, had reached out to me and said, “Hey, this is what we're building, this is what we're thinking,” and asked for some of my thoughts, and I gave them, and then he asked me to be the chair of this thing, which Matt, going back to impostor syndrome, I'm like, “Jim, I've been learning AI for three months, so I'm not an expert at doing this,” and he's like, “I can't think of anyone better. You're a student, you do take the time, you're eager to go do this, you're a practitioner, a founder, and you're going into these things. Just come and do this,” so I sort of hesitantly accepted the roll with a lot of imposter syndrome going along with it, but I've now been able to reach out and talk to some pretty amazing people that are now wanting to start forming as part of this alliance to create what is defining AI safety, and how do we think about it? So, it's going to be really exciting. It's obviously not a full-time gig. It's a sort of part-time contribution and doing my best to help move this thing along and do some great stuff.
[38:52] Matt: So, you mentioned that you left Robinhood a few months ago. Now, you're the chair of the AI safety initiative. What's next for you?
[39:02] Caleb: Right now, I'm going to continue down this path, man. I'm going to focus on keeping my education and learning, I'm going to focus on AI a lot. I have been helping what we call Day Zero entrepreneurs, founders who want to go build something but don't quite know what to build, and where do they go? How do they raise capital? How do they build their idea, learn how to validate? And I've been doing it in my spare time, helping those founders go build companies.
[39:35] Matt: Where can our listeners connect with you and follow you? What's the best place to do that?
[39:38] Caleb: LinkedIn.
[39:40] Matt: I love it. I love it.
[39:41] Caleb: It’s the only place I do social anything.
[39:44] Matt: Got to keep it all there. It is a great platform. It's a great place to have conversations, and I tell people, some people ask me “how do you do this? How do you do that?” I say it's like anything else. If you want to see a return, you have to invest in the LinkedIn platform and quite frankly, it's a great algorithm as well. So, it rewards you the more frequently you post original content, you comment. I stepped away for the last couple of months from doing it a lot because quite frankly, my full-time job has been very busy, but I tell people, “If you put the time in there, over the course of a couple of months and years, it is a great network.” I assume that's been yours as well?
[40:25] Caleb: It is because I just feel like there’s good conversations that are had they are, and there's also some accountability. LinkedIn, it's not anonymous. This is your business profile, so you have opinionated, fair, logical discussions and debates, versus, I feel, other social mediums, which could just be filled with trash, so I really appreciate LinkedIn. I think that the discussions are better and, for me, it's just the only social network I think I'll use.
[41:01] Matt: I love it. Well, Caleb, this has been a great discussion. Thanks for coming on the show.
[41:04] Caleb: Thanks, Matt.
Podcast Close: Thank you for joining us for today's episode. To find out more, please visit us at Cloudsecuritytoday.com.